Artificial intelligence (AI) is transforming nearly every facet of our lives. From facial recognition unlocking smartphones to AI-powered chatbots providing customer service, AI is rapidly becoming an indispensable tool. However, this transformative power hinges on one crucial element: data. AI algorithms are only as good as the data they are trained on.
In this data-driven landscape, data governance takes center stage. It’s the framework that ensures data quality, security, and responsible use – the bedrock upon which trustworthy and ethical AI applications can be built. But as AI continues to evolve, so too must data governance strategies.
The Challenges of Data Governance in the Age of AI
Traditional data governance practices, often focused on structured data in relational databases, face new challenges in the age of AI: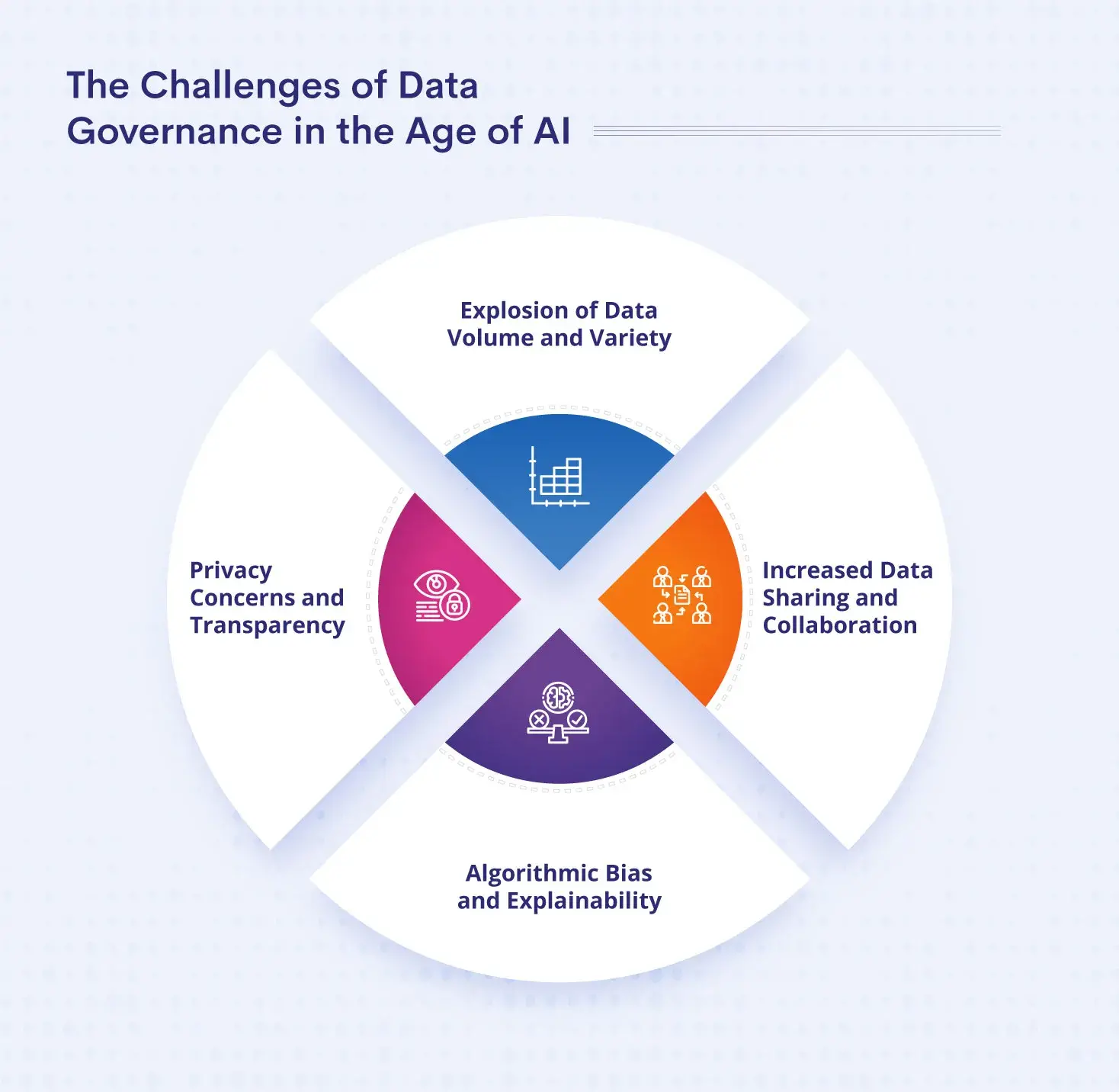
-
- Explosion of Data Volume and Variety: AI thrives on vast amounts of data, but this abundance can be overwhelming. Unstructured data, like images, text, and machine logs, requires new governance strategies for effective handling and analysis.
- Increased Data Sharing and Collaboration: AI solutions often rely on data from multiple sources, both internal and external. This necessitates robust data-sharing governance frameworks to ensure secure and responsible data exchange.
- Algorithmic Bias and Explainability: AI algorithms can inadvertently inherit biases from the data they are trained on. Ensuring data fairness and developing mechanisms for explainable AI are crucial to building trust and mitigating potential biases in AI outputs.
- Privacy Concerns and Transparency: As AI interacts with vast amounts of personal data, robust privacy controls, and transparent data collection practices are essential to maintain user trust and comply with evolving data privacy regulations.
Best Practices for Data Governance for AI Success
To effectively navigate these challenges and ensure the responsible development and deployment of AI solutions, data governance needs to adapt and follow a few best practices listed below:
-
- Focus on Data Quality and Fitness for Purpose: Data governance in the age of AI must prioritize data quality. This necessitates data cleansing, standardization, and ensuring data aligns with the specific needs of the AI application.
- Data Lineage and Traceability: Tracking the origin, transformation, and usage of data throughout its lifecycle becomes critical. This fosters transparency and allows for identifying and addressing potential biases or issues within the data used to train AI models.
- Evolving Data Access Controls: Data access controls need to be adaptable to accommodate collaboration and data sharing for AI development purposes. Granular access controls ensure the right people have access to the right data while mitigating unauthorized access and potential breaches.
- Focus on Data Privacy and Security: Data privacy regulations like GDPR and CCPA require robust security measures and user consent mechanisms for data collection and usage. Embedding data privacy considerations into the entire AI development lifecycle is essential.
- Data Governance Automation: As data volumes grow, automating data governance processes can streamline tasks like data quality checks and access control management, allowing data governance teams to focus on strategic initiatives.
The Benefits of Effective Data Governance for AI
Strong data governance practices are not just about mitigating risks; they unlock significant benefits for AI initiatives:
-
- Improved AI Model Performance: High-quality, unbiased data leads to more accurate and reliable AI models, enhancing the overall effectiveness of AI solutions.
- Increased Trust and Transparency: Robust data governance builds trust in AI, both internally within organizations and externally among users.
- Enhanced Compliance: Effective data governance helps organizations comply with evolving data privacy regulations, mitigating legal risks and potential penalties.
- Faster Time to AI Value: Efficient data governance processes and streamlined data access can accelerate the development and deployment of AI solutions, allowing companies to capitalize on their potential sooner.
The Future of Data Governance: A Collaborative Approach
Effective data governance in the age of AI requires a collaborative approach. Here are some key considerations:
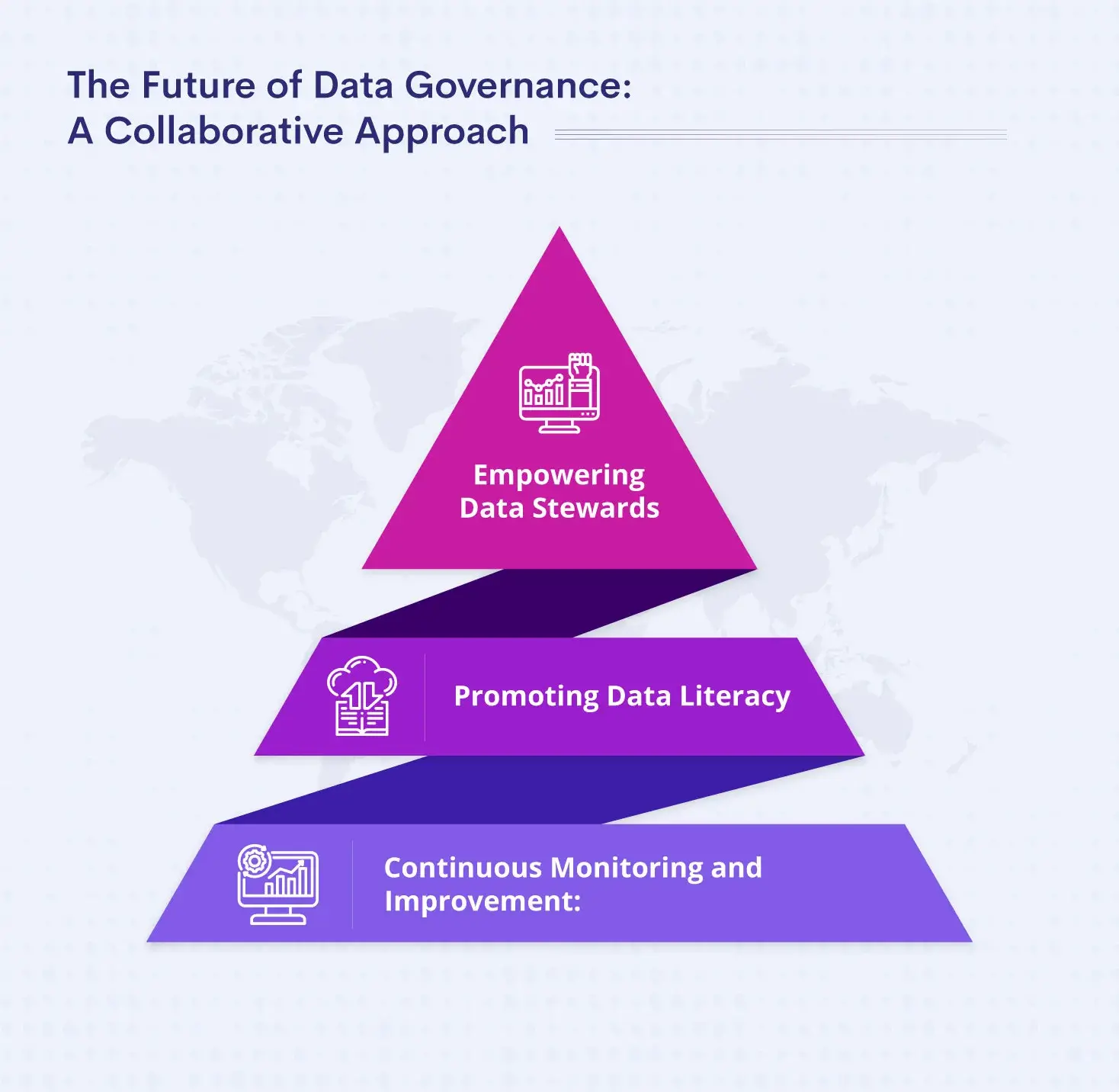
-
- Empowering Data Stewards: Data stewards, individuals responsible for data quality and compliance within specific domains, play a crucial role in AI governance. Empowering them with the necessary tools and training is vital.
- Promoting Data Literacy: A data-literate workforce fosters a culture of responsible data use and collaboration with AI systems.
- Continuous Monitoring and Improvement: Data governance is an ongoing process. Regularly monitoring data quality, performance, and user feedback allows for continuous improvement and adaptation of data governance strategies.
Conclusion: Data Governance – Empowering Responsible AI
In the era of AI, data governance transcends mere compliance; it emerges as the bedrock of responsible AI evolution and implementation. Through the establishment of robust data governance frameworks and the cultivation of a collaborative data culture, organizations can harness AI’s potential ethically, transparently, and with a keen eye on fostering positive societal change. As AI progresses, data governance will persist as a pivotal element for organizations committed to realizing the profound possibilities of this transformative technology.