As organizations strive to muddle the influence of data-driven insights, migrating to a Databricks lakehouse architecture has emerged as a popular choice. The Databricks Lakehouse platform combines the best features of data lakes and data warehouses, providing a unified analytics solution that enables efficient data processing, advanced analytics, and machine learning capabilities.
Databricks Lakehouse architecture – What makes it popular?
The Databricks lakehouse architecture integrates seamlessly with popular data sources and tools, allowing for easy data ingestion and integration. It also offers powerful data management capabilities, such as schema enforcement and data governance, ensuring data quality and reliability. With built-in support for advanced analytics, machine learning, and AI, the Databricks lakehouse architecture empowers organizations to derive valuable insights and make data-driven decisions. Overall, it revolutionizes the way data is managed and leveraged, enabling businesses to unlock the full potential of their data assets.
Eight Best Practices & Considerations for Successful Databricks Lakehouse Migration
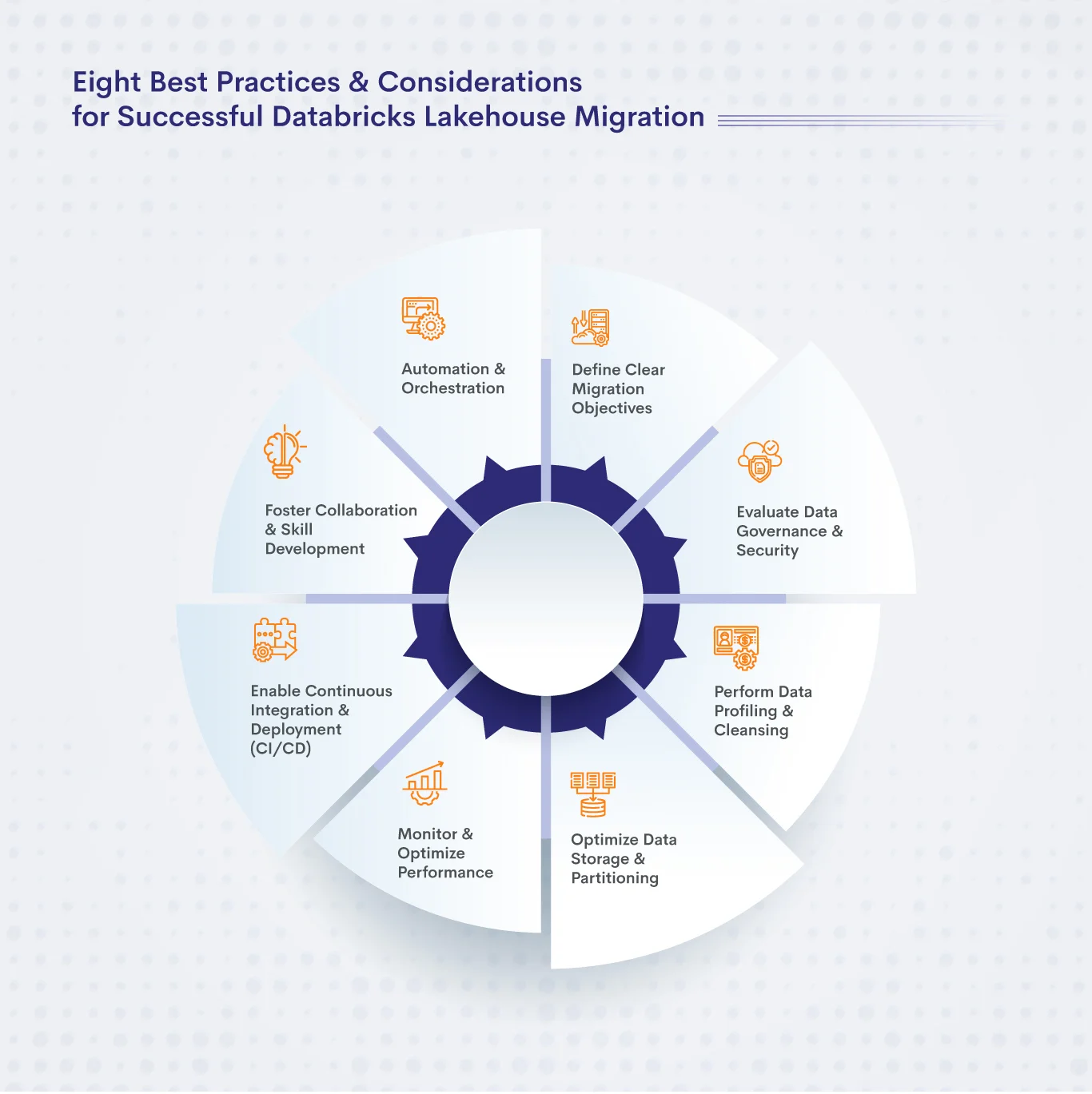
To ensure a successful migration to a Databricks lakehouse, it is important to follow industry best practices. In this blog, we discuss the key considerations and recommended practices for a smooth and effective Databricks lakehouse migration.
- Define Clear Migration Objectives: Before embarking on a Databricks lakehouse migration, clearly define your migration objectives. Assess your current data infrastructure, identify pain points and opportunities for improvement, and set measurable goals for the migration. Establishing clear objectives will help guide your migration strategy and ensure alignment with your organization’s data and analytics roadmap.
- Evaluate Data Governance and Security: Data governance and security are critical aspects of any data migration project. Prioritize a comprehensive assessment of your data governance policies and security protocols. Consider implementing fine-grained access controls, encryption mechanisms, and data classification frameworks to protect sensitive data in your Databricks lakehouse. Adhering to industry regulations and compliance standards is essential for maintaining data integrity and safeguarding your organization’s reputation.
- Perform Data Profiling and Cleansing: A successful Databricks lakehouse migration relies on the quality and cleanliness of the data being migrated. Conduct thorough data profiling and cleansing activities to identify and rectify data inconsistencies, duplicates, and inaccuracies. Leverage data quality tools and techniques to ensure that the migrated data meets the required standards for accuracy and completeness.
- Optimize Data Storage and Partitioning: Efficient data storage and partitioning strategies are crucial for maximizing the performance of your Databricks lakehouse. Leverage Databricks Delta Lake’s capabilities to optimize data storage, including advanced indexing techniques and partitioning schemes. By organizing data into optimal partitions, you can significantly enhance query performance and reduce data processing time.
- Automation and Orchestration: Automation and orchestration are essential components for a smooth Databricks lakehouse migration. By leveraging automation tools and frameworks, organizations can automate critical tasks like data ingestion, transformation, and migration, reducing manual effort and improving efficiency. Implementing orchestration workflows enables seamless coordination between various stages of the migration process, ensuring a streamlined and well-managed pipeline. This approach simplifies data integration, enhances data quality, and accelerates the overall migration timeline. Using automation and orchestration, businesses can optimize their Databricks lakehouse migration, enabling them to unlock the full potential of their data assets.
- Enable Continuous Integration and Deployment (CI/CD): Adopt a CI/CD approach to facilitate continuous integration and deployment of data pipelines and analytics workflows in your Databricks lakehouse. Implement version control systems, automated testing, and deployment pipelines to ensure consistency and reliability in deploying data and analytics artifacts. This enables faster iterations, easier rollbacks, and improved collaboration among data teams.
- Monitor and Optimize Performance: Continuous monitoring and optimization are essential to ensure optimal performance of your Databricks lakehouse. Implement comprehensive monitoring and alerting mechanisms to track query performance, resource utilization, and system health. Leverage Databricks’ monitoring capabilities, such as query plans and execution statistics, to identify performance bottlenecks and optimize resource allocation.
- Foster Collaboration and Skill Development: Successful migration to a Databricks lakehouse requires a collaborative culture and a skilled data team. Encourage collaboration among data engineers, data scientists, and analysts to foster knowledge sharing and innovation. Invest in training and upskilling programs to enhance the proficiency of your data team in utilizing Databricks Lakehouse capabilities effectively.
Conclusion:
Migrating to a Databricks lakehouse architecture opens doors for organizations to leverage unified analytics and extract valuable insights from their data. To achieve a smooth and successful migration, it is crucial to adhere to best practices. These practices encompass meticulous planning, thorough assessment of data and infrastructure, well-defined migration strategies, and seamless collaboration with a trusted Databricks migration partner. By embracing these practices, organizations can unlock the full potential of unified analytics, harness the power of data-driven decision-making, and drive innovation in their business operations.